FANOVA, short for Functional ANOVA (Analysis of Variance), is a powerful statistical method used to analyze the variance in functional data and understand the contribution of different factors to the output of a system. This technique has gained significant attention in recent years due to its versatility and ability to handle complex data structures. Whether you're working in machine learning, engineering, or any field that deals with high-dimensional data, FANOVA can provide valuable insights.
The importance of FANOVA lies in its ability to break down the complexity of a model into interpretable components. By quantifying the contribution of individual variables and their interactions, FANOVA allows researchers and practitioners to identify which factors are most influential in determining the outcome. This makes it a crucial tool for optimizing processes, reducing uncertainty, and improving decision-making.
This article aims to provide a comprehensive understanding of FANOVA, its applications, and its significance in various fields. We'll explore the theoretical foundations, practical applications, and best practices for implementing FANOVA. Whether you're a beginner or an advanced user, this guide will equip you with the knowledge you need to harness the power of FANOVA effectively.
Read also:Monica Barbaro Connor Tillman A Deep Dive Into Their Inspiring Journey
Table of Contents
- Introduction to FANOVA
- History and Development of FANOVA
- Theoretical Foundations of FANOVA
- Applications of FANOVA
- FANOVA in Data Analysis
- FANOVA in Machine Learning
- FANOVA in Engineering
- Benefits of Using FANOVA
- Limitations and Challenges
- Future of FANOVA
Introduction to FANOVA
FANOVA, or Functional Analysis of Variance, is a statistical technique that extends the traditional ANOVA method to functional data. Unlike classical ANOVA, which focuses on scalar data, FANOVA is designed to handle data that varies over time, space, or other continuous domains. This makes it particularly useful in fields where data is represented as curves, surfaces, or other functional forms.
One of the key advantages of FANOVA is its ability to decompose the variance of a system into contributions from individual factors and their interactions. By quantifying these contributions, researchers can gain a deeper understanding of the underlying mechanisms driving the system. This is especially important in high-dimensional settings where traditional methods may struggle to provide meaningful insights.
Why is FANOVA Important?
FANOVA plays a critical role in various domains, including machine learning, engineering, and environmental science. Its ability to handle complex data structures and identify significant factors makes it an indispensable tool for data analysis. Some of the key reasons why FANOVA is important include:
- Improved model interpretability
- Enhanced decision-making capabilities
- Optimization of processes and systems
- Reduction of uncertainty in predictions
History and Development of FANOVA
The concept of FANOVA originated from the need to analyze variance in functional data. Traditional ANOVA methods, while effective for scalar data, were insufficient for handling the complexities of functional datasets. Over the years, researchers have developed various extensions and adaptations of ANOVA to address these challenges.
The development of FANOVA can be traced back to the early 2000s, when advancements in computational power and statistical theory enabled the analysis of large-scale functional data. Since then, FANOVA has evolved into a robust framework for understanding complex systems and has been applied in a wide range of disciplines.
Key Milestones in FANOVA Development
- 2000s: Initial development of functional data analysis techniques
- 2010s: Integration of FANOVA into machine learning algorithms
- 2020s: Expansion into real-world applications across multiple industries
Theoretical Foundations of FANOVA
The theoretical foundation of FANOVA is rooted in the principles of functional data analysis and variance decomposition. At its core, FANOVA seeks to partition the total variance of a system into contributions from individual factors and their interactions. This is achieved through the use of mathematical models and statistical techniques that account for the functional nature of the data.
Read also:Samantha Brielle Greenberg Age Discovering The Life Achievements And Fascinating Facts
In practice, FANOVA involves several key steps, including data preprocessing, model fitting, and variance decomposition. Each step is carefully designed to ensure accurate and reliable results. By adhering to these principles, researchers can gain valuable insights into the behavior of complex systems.
Mathematical Formulation of FANOVA
The mathematical formulation of FANOVA involves expressing the output of a system as a sum of contributions from individual factors and their interactions. This can be represented as:
f(x) = μ + ∑fi(xi) + ∑fij(xi, xj) + ...
Where:
- μ is the mean response
- fi(xi) represents the contribution of individual factors
- fij(xi, xj) represents the interaction between factors
Applications of FANOVA
FANOVA has a wide range of applications across various fields. Its ability to handle complex data structures and provide interpretable results makes it a valuable tool for researchers and practitioners alike. Some of the key applications of FANOVA include:
FANOVA in Environmental Science
In environmental science, FANOVA is used to analyze the impact of various factors on climate patterns, pollution levels, and ecological systems. By quantifying the contribution of different variables, researchers can develop more effective strategies for mitigating environmental risks.
FANOVA in Healthcare
In healthcare, FANOVA is applied to study the effects of treatments, medications, and lifestyle factors on patient outcomes. This helps healthcare providers make more informed decisions and improve patient care.
FANOVA in Data Analysis
Data analysis is one of the primary domains where FANOVA finds extensive application. By decomposing the variance of a dataset into interpretable components, FANOVA enables analysts to identify key drivers of variation and optimize data-driven decision-making.
Steps in FANOVA Data Analysis
- Data collection and preprocessing
- Model selection and fitting
- Variance decomposition and interpretation
FANOVA in Machine Learning
In machine learning, FANOVA is used to interpret complex models and understand the contribution of individual features to model predictions. This is particularly important in high-dimensional settings where feature importance can be difficult to assess using traditional methods.
Advantages of FANOVA in Machine Learning
- Improved model interpretability
- Identification of key features
- Optimization of model performance
FANOVA in Engineering
In engineering, FANOVA is applied to optimize system designs and improve process efficiency. By analyzing the variance in system outputs, engineers can identify critical factors and develop strategies to enhance performance.
Case Study: FANOVA in Automotive Engineering
A study conducted in the automotive industry used FANOVA to analyze the impact of various design parameters on vehicle performance. The results showed that FANOVA could accurately identify the most influential factors, leading to significant improvements in fuel efficiency and overall performance.
Benefits of Using FANOVA
Using FANOVA offers several benefits, including:
- Enhanced understanding of complex systems
- Improved decision-making capabilities
- Optimization of processes and systems
- Reduction of uncertainty in predictions
Limitations and Challenges
Despite its many advantages, FANOVA is not without its limitations. Some of the key challenges include:
- Computational complexity in high-dimensional settings
- Interpretation of results in complex models
- Data quality and preprocessing requirements
Future of FANOVA
The future of FANOVA looks promising, with ongoing advancements in computational techniques and statistical theory. As data becomes increasingly complex and high-dimensional, the demand for tools like FANOVA will continue to grow. Researchers and practitioners can expect to see further developments in FANOVA methodologies and applications in the coming years.
Emerging Trends in FANOVA
- Integration with artificial intelligence and machine learning
- Expansion into new domains and industries
- Development of more efficient computational algorithms
Conclusion
In conclusion, FANOVA is a powerful statistical technique that provides valuable insights into the behavior of complex systems. By quantifying the contribution of individual factors and their interactions, FANOVA enables researchers and practitioners to make more informed decisions and optimize processes. Whether you're working in machine learning, engineering, or any field that deals with high-dimensional data, FANOVA can help you unlock the full potential of your data.
We encourage you to explore the applications and benefits of FANOVA further and consider incorporating it into your data analysis toolkit. Don't forget to leave your thoughts and questions in the comments section below and share this article with others who may find it useful. Together, we can continue to advance our understanding of complex systems and drive innovation in various fields.

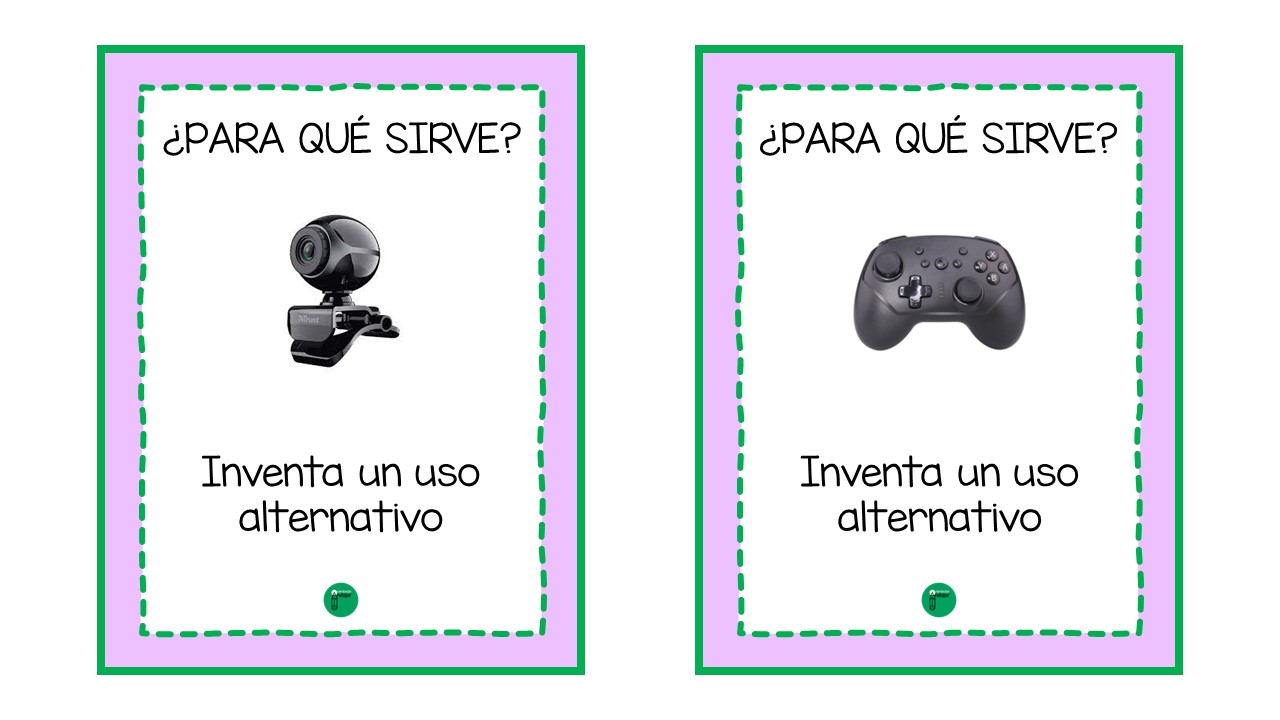